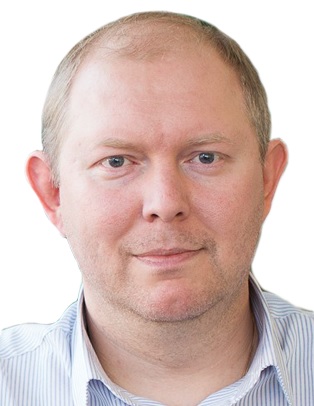
By Sergey Soldatov
Sergey Soldatov is the head of security operations center at Kaspersky. He is an information security professional with over 20 years of experience in different fields of IT and information security.
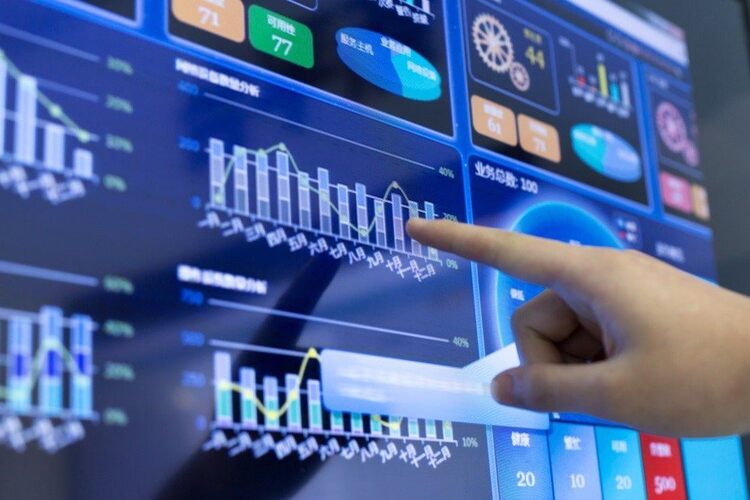
It’s no secret that cybersecurity is very expensive. In 2021 the average IT Security Budget amounted to US$11.4 million for enterprises and US$267k for SMBs.
At the same time, insufficient security measures can lead to disastrous consequences and significantly affect a business’ reputation and budget. For instance, a data breach costs a large organization $927K, on average, and up to half of the company’s customers can be lost following such an incident.
This situation leads to a dilemma – on the one hand, companies would be happy to find a solution to reduce their cyberdefense budget, while on the other, the cost of an error when introducing new and cheaper tools in this field is way too high. One answer is automated incident prevention – a good solution that can reduce costs and eliminate the human mistake factor. In addition, people tend to trust AI more than a human colleague.
However, in practice, effective cyberprotection is only possible with a combination of automated solutions and human effort. Why is that?
First of all, cybercrimes are committed by human beings. Like all of us, they can make decisions based on a mix of different cognitive processes and quickly adapt to the environment. Attackers constantly come up with new ways to bypass security systems, invent and implement new sophisticated attack tactics and actively use people’s weaknesses to gain access to a company’s infrastructure. Even the most sophisticated AI can’t combat the variety of malicious activities out there because it works on the basis of previously acquired and learned experience.
Below we consider several cybersecurity practices that require human involvement.
Detection of complex threats
Even the most carefully tuned sensors can’t detect previously unknown malicious activities. This is because such attacks usually consist of a series of separate and legitimate actions that could easily be confused with system administrator or common user actions. Fileless attacks, heavy use of LOLBAS tools, runtime encryption, downloaders and packers – are all widely used to help attackers bypass security solutions and controls.
AI that analyses telemetry from sensors also has limitations: it can’t collect and process all possible data or actions that occur at different times. Even if that was possible, there is another issue – situational awareness. This term refers to the availability of information about all the processes currently taking place in the infrastructure. A simplest example is when an AI observes what it believes to be a human-driven APT but in fact turns out to be a dedicated employee conducting research. This can only be uncovered by contacting the customer, for example, by phone. Situational awareness is crucial to differentiate true incidents from false-positive alerts such as this, no matter if the alert logic is based on a particular attack technique behavior pattern or anomaly analysis.
This doesn’t mean that AI is ineffective in terms of threat detection. Infact it can successfully combat 100% of known threats and, when properly configured, can significantly reduce the burden on analysts.
At Kaspersky we have developed a machine learning (ML) analyst for our MDR service. It is a supervised ML algorithm that uses labeled historical data for the primary classification of alerts as false or true positives. All alerts from protected assets are initially processed by it. A little more than a third of activity the algorithm classifies comes out as a false positive and anything that exceeds the threshold or specified filtration rule is sent to the security analyst team for examination. Someone on that team then evaluates each alert, using additional methods and data suitable for the particular case which may not have been used by the AI. After the human analysts find a way to solve the problem, they share this experience with the ML analyst so the next case won’t be a challenge for the AI.
This joint force approach requires special skills, high-grade analyst experience and constant algorithm adjustment. The good news is that it enables security teams to tackle even the most dangerous situations, such as the famous PrintNightmare vulnerability exploitation or MuddyWater APT attack and share those valuable detection scenarios with others.
When identifying new threats, proactive manual threat hunting is also required. This allows a security team to hunt out threats that are lying undiscovered but still active within the company’s infrastructure. Proactive threat hunting allows an organization to identify current cybercriminal and cyberespionage activity in the network, understand the reasons behind these incidents and the possible sources, and effectively plan mitigation activities that will help avoid similar attacks.
To sum up, analysts have to constantly adjust and retrain the AI-based algorithm, enabling it to detect new threats as well as test the efficiency of the improvements.
Advanced security assessments
Assessments are crucial to gain a detailed perspective of a company’s cybersecurity readiness. Of course, there are automated solutions designed for this. For example, a commonly known vulnerability assessment can help to discover publicly-known vulnerabilities among a strictly defined set of systems. Still, this service uses a database of already known security issues but can’t test security system resilience towards sophisticated attacks and unconventional adversaries’ behavior.
To ensure that the company is able to protect itself, more advanced assessment processes should be implemented. For example, services that can actually simulate a cyberattack, such as penetration testing and red teaming, that are mostly manual and based on a specialist’s knowledge and experience. These approaches use a mix of techniques, tactics and procedures and adjust to the company’s specific cyber defense capabilities, imitating the real behavior of attackers.
Security awareness
Studies indicate that the average organization faces over 700 social engineering attacks each year. Moreover, weak passwords and phishing emails are still among the top initial attack vectors. Attackers keep an eye on trends and act like good psychologists. You can be sure that each trigger – from the pandemic to Kanye West’s new album – will be used by adversaries to attract the attention of a potential victim through phishing emails and malicious websites to achieve their goals.
While cybercriminals are inventive, an organization’s defense team can’t completely withdraw themselves from the security awareness processes. A company’s employees need to have a clear understanding of the importance of cybersecurity policies as well as the consequences of their actions. That is why it is not enough to simply develop an awareness manual or test that is only used for onboarding new team members. The IT security team should keep an eye on the relevance of their security education and invent new and non-standard approaches to deliver crucial information to their colleagues. Another way of solving this problem is to outsource these activities to a professional security awareness training team that ensures information is regularly updated and delivers an engaging learning experience.
Nobody is saying that security teams should abandon automatization or fight against cybercriminals with their “bare hands”. Particularly as attackers strive to be as effective as possible, often resorting to automated solutions, using machine learning to gather information about potential targets, brute force passwords, and find vulnerabilities through fuzzing, DDoS attacks, malware creation and so on.
Instead, the truth lies somewhere in the middle. Only a smart mix of automated solutions with human creativity, skills and control can ensure comprehensive cyber defense.
* The views expressed herein are strictly the personal opinion of the writer and do not necessarily represent the views of YamCha Time.